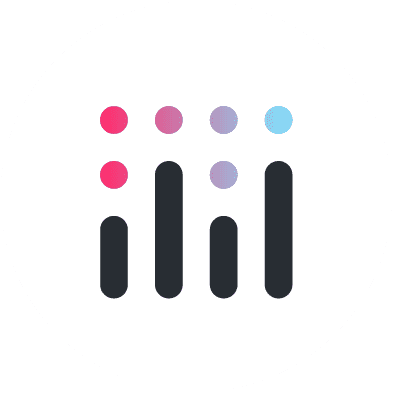
Plotly
October 17, 2023 - 5 min read
How Pharma and Life Sciences Are Benefiting From AI Applications
In 2022, 35% of organizations incorporated AI into their business, with a further 42% expressing interest in the application (IBM, 2022). For a data focused industry, such as pharma, healthcare, and life sciences, streamlining data science would be a welcome addition. And this is where AI shines. In this article, we will explore how AI is benefiting employees and patients alike, which obstacles still need to be overcome, and what the future holds.
How AI is shaping healthcare
Discovering and manufacturing new drugs is a notoriously laborious and costly process. AI has become vital in saving both time and money:
- First, teams need to identify which macro-molecules the therapeutic agents need to target. AI can employ multiple techniques to process large amounts of data, speeding up this process dramatically.
- With this target in sight, research teams then need to filter the successes from the failures. This is achieved through High Throughput Screening (HPS), an automated process of testing millions of samples for the required biological activity on the cellular level. Pairing this process with Virtual Screening (VS), the process of testing how compounds from a chemical library react to the target, leads to a higher hit rate.
- Once the team identifies what will work, AI can even design drug molecules by predicting the target protein structure and drug-protein interactions.
- At the final stage of clinical trials, AI can utilize Predictive Modelling to analyze clinical trial reports, determining why they were successful or not, and use this information to predict the outcome of future trials, effectively learning from past mistakes.
Here are a few additional ways in which AI is making an impact on healthcare:
Personalized Medicines
AI is opening up the exciting new field of Personalized Medicines (PM) which allow treatments to be specially tailored to individual patients instead of relying on the "one size fits all" methods currently used:
- Diagnosing the problem is difficult purely based on images, but complex Machine Learning (ML) models can be taught to analyze an image, such as a brain scan, to look for anything from a stroke to Alzheimer's.
- For many diseases, cancer in particular, getting an early diagnosis could mean the difference between life and death. Algorithms can be created to allow ML to identify patients most at risk from such diseases (known as Early Disease Detection). In 2017, a study conducted on patients at risk of stroke used an AI algorithm comparing symptoms to medical history to successfully place patients into the early detection stage with an accuracy rating of 87.6% (Jiang, et. al., 2017).
- Biomarkers, indicators of a biological state in reaction to disease, are believed to be the key elements of PM. AI models can be used to identify biomarkers often missed by a human.
- Precision medicines is a term which describes taking the individualizing variables of the patient, including genes, environment and lifestyle, into consideration when treating and preventing illness. Combining AI with precision medicines will revolutionize healthcare as we know it.
Individual health care
- An Electronic Medical Record (EMR) is a digitized version of a patient’s medical history. Adopting AI into EMRs will be key to many of the innovations described above. However, it is important to note that, in order to do this, much of the unstructured data will need to be standardized in order to be read by AI models.
- Natural Language Processing (NLP) allows text documents to be converted into datasets which can be read by ML algorithms. This will allow the ML model to quickly analyze a patient's medical record, identifying the most urgent risks and actions, as opposed to a doctor leafing through various outpatient letters and test results, dramatically speeding up the process of diagnosing the patient.
- Chatbots are computer programs which simulate and process human conversation. Recent studies have shown that chatbots have many applications to aid the treatment of cancer including diagnosis, delivering post treatment results, monitoring the patient's progress and even offering support.
- Predictive Analysis (PA) combines mathematics and statistics to make predictions. By processing large amounts of data, both historical and real time, PA can make valuable predictions regarding the health of both individual patients and the public as a whole. This can be used to dramatically improve hospital efficiency.
Genomics
The study of our genes and their interactions (known as Genomics) is a very important field regarding many of the advancements we have discussed. When you consider that 1g of DNA can hold up to 215 million gigabytes of information, AI is a vital tool:
- The use of Deep Learning (DL) and alignment technologies allows researchers to map DNA sequences with astonishing speed.
- The development of personalized treatments will rely heavily on efficient genomic data interpretation. DL’s ability to combine knowledge from scientific literature with the results of screenings to predict how genetic variation will affect disease presentation and the most effective therapeutic method.
- Combining AI with systems like CRISPR/Cas9, a genome editing tool, will allow for the alteration of genomes in cancerous tumors and modify genetic mutations which could lead to cancer or other genetic diseases.
Challenges With AI in Pharma and Life Sciences
AI's impressive data-processing speed is its greatest strength, but it comes with drawbacks. AI is only as good as the data it is fed. If that data is not of high quality, the outcome will be poor with potentially harmful consequences to the patient. This is of great concern considering health data is spread across multiple systems and organizations where it can easily become fragmented and disorganized.
It can also be difficult for healthcare professionals to understand how an AI has reached a particular conclusion, this is known as the “black box” problem. Through the integration of Explainable AI (XAI) we can avert this issue by helping build user confidence.
When it comes to patient data, inevitably the issue of confidentiality must be raised. Any data acquisition must comply with various rules governing consent and identity protection, a potential logistical headache. The use of privacy-enhancing-technologies (PETs) and data masking techniques can be used to protect patients' data.
One of the main fears is that AI will eventually replace humans. In the medical industry this is of particular concern as the lack of empathy and compassion that separates us from machines can leave patient’s hesitant to engage with such practices. It is therefore important to emphasize that AI tools are meant to support medical professionals and not replace them. This can be accomplished through training employees in the applications of AI, giving them more confidence working with such systems.
What the future holds
The combination of AI and automation through robotics is a key stage in improving the manufacture of drugs and quality control.
Clinical Decision Support (CDS) systems are computerized systems which enhance medical decisions based on clinical knowledge and patient information. The use of AI based CDS (AI-CDS) would be a key component to incorporating predictive analysis into hospital management as well as unlocking the full benefits of EMRs.
Drug repurposing is the practice of discovering new uses for existing drugs as opposed to creating new ones from scratch. By utilizing the data processing techniques discussed earlier, AI can help speed up the extensive screening process required for this method to be a success.
Dash Enterprise allows the streamlined creation of data apps employing custom analytics and interactive data exploration to allow access to the vast amounts of information demanded by the Pharma and Life Sciences industries. This data can be easily visualized through 3D imaging and graph plotting while Continuous Integration and Continuous Delivery (CI/CD) pipelines ensure the software always runs efficiently and can be easily modified. To learn more about using AI to push the boundaries of modern medicine, contact us for a demo.