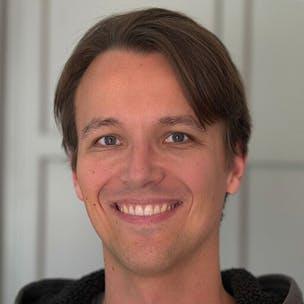
Robert Claus
April 23, 2025
uv Package Manager Quirks a Year Into Adoption
Here at Plotly, we first and foremost cater to the Python community. Effectively anything that needs to interact with our community’s code is written in Python to be compatible with our open source libraries, Plotly Express and Dash. This means we have a lot of standalone Python projects and many custom libraries internally.
As we worked on these Python projects over the last year, we adopted an unassuming tool called 'uv'. Our team has been gradually falling more and more in love with uv as a tool to improve our Python working environments. As useful as it is, this package manager comes packaged with a few quirks of its own. This article will explore uv, its surprising quirks, and the incredible value it brings to our workflows.
What is the uv package manager?
The uv Python package manager is effectively a replacement for venv and pip rolled into one. It provides almost perfect backwards compatibility with pip — so adoption is generally considered trivial. The speed it runs at has blown us away and the convenience features for managing projects are amazing.
Some core features in uv have many benefits and actually enabled product architectures we wouldn’t have considered without it:
- The default sync / lock behavior it provides without additional libraries
- The ability to install arbitrary Python versions as part of environment setup
- The ability to reliably sync environments when running a project
- The ability to run scripts in ephemeral environments because installing is so fast
Over time, we’ve successfully replaced many complex Python CI pipelines and setup scripts with clean pyproject.toml files and uv run xyz. This has saved us hundreds of lines of code, improved test setup times by an order of magnitude, and dramatically simplified managing those projects.
That being said, using uv in conjuncture with a decade of Python code hasn’t come without its challenges. While we adopted uv for greenfield prototypes fairly early, we actually held off on retrofitting old legacy projects. This was painful since we knew those projects would benefit the most from uv, but we also knew rolling it out to production projects slowly was the responsible approach.
Ultimately this care paid off and we caught a number of issues along the way.
Surprises using the uv package manager
Since uv has fundamentally changed how we think about packaging and shipping Python projects, we wanted to share our favorite examples of issues that surprised us.
index-strategy
Almost immediately we discovered that the behavior of one specific flag: `--extra-index-url` was different than it was with pip.
This was unfortunate for us because we always recommend this pip flag to our Enterprise customers when connecting to our Enterprise package index for the libraries included with Dash Enterprise, including Dash Design Kit and Dash Snapshot Engine. The goal of providing these packages in a custom index has always been to make installing them as simple as possible, so it was surprising to find that our existing pip commands didn’t work with uv.
For example, our users would write a requirements.txt file like:
plotlydashdash-design-kit
In this example, pip would pick up the dash-design-kit from the custom index and fall back to PyPI for the public ones.
However, we quickly discovered the uv had implemented a different default: Running `uv pip install -r requirements.txt –extra-index-url=mypackages.com` would generate an error saying the public packages `plotly` and `dash` couldn’t be found on mypackages.com. uv treats the extra index as the only index by default. This means any packages not duplicated in our private index would fail to install by default.
For new projects, this new behavior provides real protection for end users by mitigating a whole class of dependency attacks. Additionally, the change is only a problem with basic requirements.txt files - a pyproject.toml file like `uv init` generates can explicitly handle specific indexes for specific packages. Interestingly, pip may also move to this default in the future since it is now the Python community’s recommendation. However, for established pipelines and customers it did present a problem.
Last spring, after much discussion on GitHub, uv introduced index-strategy to support installation from multiple indices. This still means setting this in any CI pipeline that depends on this behavior. It has hindered adoption amongst our customers, though our Customer Success team has been encouraging use of uv for its simplified environment and Python management tools, which are some of the highest priority issues for our customers. Many of our users still encountered the same issue as they expected uv pip to be a drop-in replacement for `pip` - and in this particular case it isn’t.
compile-bytecode
One of Plotly’s core open source libraries is Dash — a framework for building full stack applications with front end interactions directly in Python. This means our testing pipelines generate hundreds of standalone Python applications per day. We thought this would be a great opportunity to leverage uv for performance gains! However, we quickly learned that our environment setup bottlenecks weren’t quite what we thought.
As an example, take a simple Python script that imports Plotly’s open source Dash library:
import dashprint("Done!")
In a traditional pip-enabled workflow, this might pair with a requirements.txt that just contains that one library. We might then set up a virtual environment, install the dependencies, and run the script:
python3 -m venv .venvsource .venv/bin/activatepython -m pip install --no-cache-dir -r requirements.txtpython app.py
On my M3 Macbook Pro, the venv and pip version takes approximately 15 seconds to set up, 0.4 seconds to run the first time, and 0.2 seconds to run after that.
In uv we would have three options for running the script with dependencies.
- Use uv pip similar to pip directly — except that it would handle environment setup for us.
- Replace the requirements.txt with a pyproject.toml - which uv will then respect and install automatically when we run the script with uv run.
- Add dependencies within the script file itself.
In this example, let’s use a pyproject.toml since it scales best to larger projects. That pyproject.toml file would simply replace our requirements.txt and might look like this:
[project]name = "dash-app"version = "0.1.0"requires-python = ">=3.13"dependencies = ["dash"]
We could run the script in uv and have it take care of installing the dependencies automatically, but to easily time the steps we can also split it into two steps similar to pip:
uv sync --no-cacheuv run --no-sync app.py
What we find is that this takes about four seconds to install dependencies, and an additional second to run the first time. On subsequent runs it takes 0.2 seconds: For close readers, that’s identical performance to pip.
uv installs the dependencies incredibly fast, but then the script itself takes almost twice as long as pip on the first start. What’s going on? Why is uv’s first start time slower than pip’s?
The answer is that Python source code is compiled to bytecode. This takes time! As it turns out, pip does this by default during the install step, whereas uv lets the compiler compile to bytecode dynamically the first time the library is used. This has the benefit of only compiling the libraries that are actually used, which can be really useful when your project has hundreds of dependencies-of-dependencies that are never called. This is common in data science projects as a single script is unlikely to use all functionality in a library like numpy.
Both uv and pip offer flags to switch this behavior on or off. When we do so, we get the following timings:
Category
Install (s)
First Run (s)
Second Run (s)
pip (default)
15.2
0.4
0.2
uv (precompile)
7.1
0.4
0.2
pip (no precompile)
6.4
1
0.2
uv (default)
3.9
1
0.2
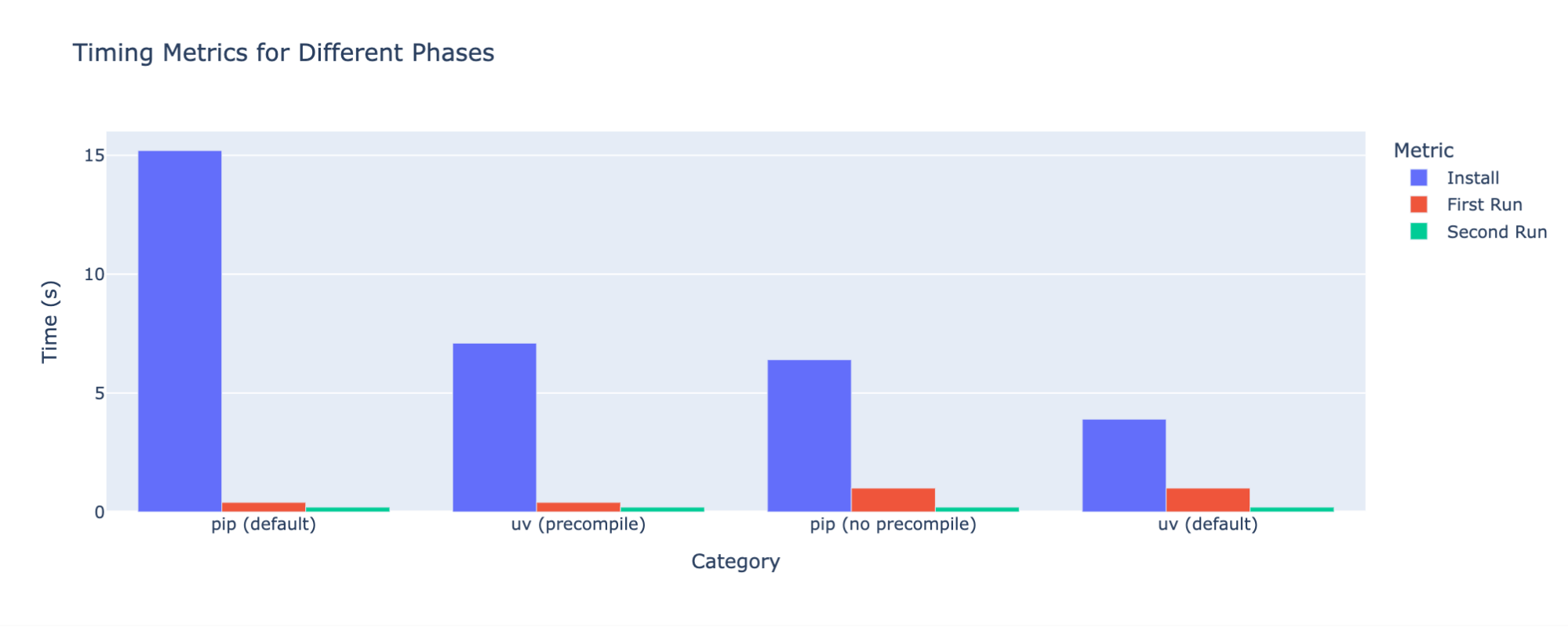
Swapping the pre-compile flags flips the pip first run to one second, and the uv first run to 0.4 seconds. That makes it pretty clear that the discrepancy is caused by this compilation step, not uv and pip themselves. We can see that uv still performs significantly faster than the equivalent pip flags.
However, this default has real life consequences. Let’s consider an example: Say you are dynamically scaling Python workers in a SaaS production environment. You may build your image once but scale up and down hundreds of workers per day. You expect uv to be a drop in replacement — and it saves 100s of seconds at build time — but then you discover your worker startup latency went up by 50%. This could be even worse in a serverless environment where you could be starting from a fresh image for every task. In reality, you just need to set one flag in your build pipeline, but that only helps you if you’re aware of the nuance.
Ultimately uv’s decision is the right one for Plotly’s community and customers as it speeds up scripting and most common workflows, but is a noteworthy quirk developers should be aware of.
whl file names
After years of developing Python build pipelines, we have a huge backlog of legacy projects and scripts. Some are more mature than others, but ultimately all go through a manual pre-release regression testing process to protect our users from unexpected bugs. Many of our older pipelines do this by building a release .whl file and renaming it with an alpha naming convention.
This isn’t best practice today, and most of our newer pipelines explicitly version our alpha releases. However, since these are some of our older libraries they are also our slowest in tests and other automations, we expected switching them over to uv would give us a boost effectively for free.
As it turned out, switching to uv completely broke the build process for these older libraries because it blocks installing any .whl file whose name didn’t match the underlying library metadata. So if a .whl was built as my-library-v1.0, but the file name was my-library-v1.0-alpha.whl, uv would prevent installation.
This backwards compatibility at first puzzled us as an odd break from pip. However, the real twist was that this wasn’t actually uv specific: Upgrading pip led to the same issue.
One natural side effect of actively using two compatible tools across legacy projects is that you start running into breaking changes in one tool before the other. Moreover, you tend to blame the newer tool for any issues. In this case, Python recognized in PEP 0708 that it was too easy to change package filenames and trick users into installing them.
Since then, we’ve been very careful to really evaluate whether any uv issues we encountered were actually caused by uv, or a change in Python that we just hadn’t encountered yet.
UV earned its place in our Python workflows
While we’ve run into some of the quirks of uv over the last year, it’s really only because it works so well that we have been pushing it on a wide range of projects. We have received a tremendous amount of value from uv in our workflows. It’s been valuable to the point that we are building new products that leverage it, and in a short span of time it truly has fundamentally changed the way we work on projects by making environment creation clean, trivial, and near-instant.