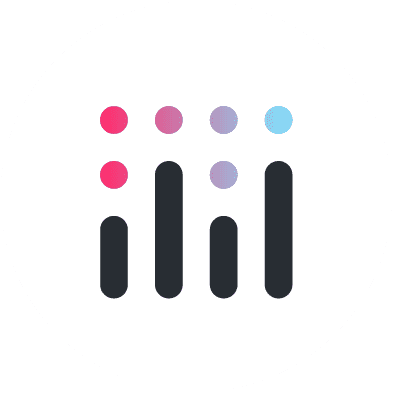
Plotly
September 29, 2023 - 5 min read
Introduction to Data Science in Finance
Information is power. That’s an aphorism usually attributed to 16th century philosopher and politician Sir Francis Bacon, and it is the root of a wide variety of strategies we follow in life and in business to this day.
Data science is the collection and analysis of information and it is used in finance to make more rigorous strategic decisions. There is more information about buyer behaviour than ever in the tech age, relating not only to who purchases what but also to browsing patterns, time spent comparing different items, feedback and discussions on social media and plenty more.
The business that makes the best use of all this information places itself at an advantage against the competition. But it also makes better decisions, directing investment dollars into the most profitable strategies and ultimately having the optimum effect on the bottom line. As such, the importance of data science in the financial sector cannot be over stated.
Data science is a discipline in itself, and a financial data scientist applies specific data science techniques to relevant data relating to customers and finance. This data can come from a wide array of sources including internal analytics tools, retail and investment banks, public domain financial tools, hedge funds and other sources. As well as gathering the data, financial data scientists build the necessary statistical analysis processes, models and techniques that will allow them to understand what the data is telling them, draw insights and make informed recommendations for short, medium, and long term strategy.
Stages of data science
Like any scientific procedure, there are different steps in the data science. They can be summarized as follows:
- Data Collection and Aggregation - data can come from diverse sources, ranging from purely financial numbers, such as sales figures, to word data such as customer reviews. So first it needs to be gathered and separated into what we can picture as separate piles.
- Data Cleaning and Preprocessing - the piles of data then need to be prepared for analysis. This might involve a variety of processes, such as putting all the data into a particular format or feeding it into a database so that it can then be meaningfully analyzed.
- Data Analysis and Exploration - this is when the analyst starts to really get to work on the data, examining what is there and seeing what immediate conclusions can be drawn, for example an increase or decrease in sales for a particular item.
- Machine Learning and Predictive Modeling - anyone with a knowledge of the business and a basic comprehension of the data can probably perform the initial analysis. The next step is to explore what the data tells us about the future. Predictive modelling demands a mixture of assumptions, but most of these are based on clues in the existing data and feeding these in to known or highly probable future factors, such as a seasonal change in demand or the impact of some forthcoming regulation.
- Data Visualization and Communication - in some ways, this is the most important step of all. Data science does not, in itself, produce concise conclusions and recommendations. The data scientist must present the results in a way that will make both the recommendations and their bases clear to a non-technical audience such as c-suite managers, shareholders or investors.
Examples of Data Science in Finance
Data science has a role to play across several areas of finance. Here we look at three specific examples:
Risk Assessment and Management
- Credit risk modelling - to understand the inherent risk from creditors and potential future impact of bad or doubtful debts.
- Fraud Detection and Prevention - identifying unusual or otherwise “red flagged” transactions that could indicate fraud or other dishonest activities.
- Market risk analysis - understanding broader trends in the market and risks therein, such as economical, political, competitive, etc.
Personalized Financial Services
- Robo-Advisors and Algorithmic Trading - these work from a rage of market indicators and signals to advise traders on when to buy and sell. They can even undertake the actual trading according to predefined parameters.
- Tailored Financial Recommendations - these could relate to investments and portfolios, pensions and the buying and selling of other financial assets.
Customer Behavior Analysis
- Customer Segmentation - identifying customer groups and their characteristics, communication preferences, buying habits, etc.
- Churn Prediction - identifying customers who are likely to desert or cancel a subscription
- Cross-Selling and Up-selling Strategies - identifying existing customers who are likely to be interested in purchasing additional products or services
Case Studies
Plotly's Dash Enterprise platform enables data scientists to deliver insights for some of the best known and most trusted businesses in the world. Here are just two examples:
Standard and Poors used a Dash app to leverage Natural Language Processing (NLP) for environmental, social, and governance (ESG) scoring. They also built a daily and quantifiable time series sentiment based on real-word mandatory disclosures, and used a range of Dash Enterprise features such as the Snapshot Engine to accelerate value from the models to decision makers. You can hear about the project direct from S&P’s Senior Director of Financial Engineering Moody Hadi via this video.
Intuit has 100 million customers worldwide and is always looking for ways to improve their efficiency and consistency. This drove the decision to adopt Python and Dash Enterprise for building interactive experimentation tools, and the net result was to reduce experiment runtimes by 50%. Although it only took a couple of scientists a couple of weeks to create an actionable version, it started to pay dividends straight away. It can be used across the full suite of Intuit services and has so far saved the client dozens of analyst hours.
Challenges and Limitations of Data Science in Finance
Data science opens up new worlds of opportunity for making smarter strategic decisions to optimize the bottom line and gain competitive advantage. But it is not without risks of its own.
When personal customer data is used, this must be treated in accordance with the appropriate data protection laws and information. That means ensuring adequate controls are in place to control its storage, access and use. Regulators have not been shy over recent years to take large organizations to task over breaches. Fines can be considerable, as can the reputation damage caused by adverse publicity.
There is also risk associated with the analytical process itself. The old adage of “garbage in / garbage out” holds true here, so there needs to be a process in place to ensure data quality and accuracy.
Finally, it is important not to get carried away with data analysis. Algorithms can give astute and accurate predictions, but they are not fool-proof. A blinkered approach can lead to disaster, so data science should only be one part of a finance department’s strategic toolbox.
Future Trends
Data science is still in its relative infancy, and its applications within finance will grow in multiple directions in the months and years ahead.
Expect to see new applications for Natural Language Processing, especially in areas like fraud prevention and detection as the technology improves and gets smoother. Predictive analytics is also only in the early stages, ,and in the years ahead are likely to be far more accurate and granular in flagging issues like credit risk.
Data science will have a more important role than ever as it evolves and adapts.