Dropdown Menus in Python
How to add dropdowns to update Plotly chart attributes in Python.
New to Plotly?
Plotly is a free and open-source graphing library for Python. We recommend you read our Getting Started guide for the latest installation or upgrade instructions, then move on to our Plotly Fundamentals tutorials or dive straight in to some Basic Charts tutorials.
Methods¶
The updatemenu method determines which plotly.js function will be used to modify the chart. There are 4 possible methods:
"restyle"
: modify data or data attributes"relayout"
: modify layout attributes"update"
: modify data and layout attributes"animate"
: start or pause an animation
import plotly.graph_objects as go
import pandas as pd
# load dataset
df = pd.read_csv("https://raw.githubusercontent.com/plotly/datasets/master/volcano.csv")
# create figure
fig = go.Figure()
# Add surface trace
fig.add_trace(go.Surface(z=df.values.tolist(), colorscale="Viridis"))
# Update plot sizing
fig.update_layout(
width=800,
height=900,
autosize=False,
margin=dict(t=0, b=0, l=0, r=0),
template="plotly_white",
)
# Update 3D scene options
fig.update_scenes(
aspectratio=dict(x=1, y=1, z=0.7),
aspectmode="manual"
)
# Add dropdown
fig.update_layout(
updatemenus=[
dict(
buttons=list([
dict(
args=["type", "surface"],
label="3D Surface",
method="restyle"
),
dict(
args=["type", "heatmap"],
label="Heatmap",
method="restyle"
)
]),
direction="down",
pad={"r": 10, "t": 10},
showactive=True,
x=0.1,
xanchor="left",
y=1.1,
yanchor="top"
),
]
)
# Add annotation
fig.update_layout(
annotations=[
dict(text="Trace type:", showarrow=False,
x=0, y=1.085, yref="paper", align="left")
]
)
fig.show()
Update Several Data Attributes¶
This example demonstrates how to update several data attributes: colorscale, colorscale direction, and line display with the "restyle" method.
import plotly.graph_objects as go
import pandas as pd
# load dataset
df = pd.read_csv("https://raw.githubusercontent.com/plotly/datasets/master/volcano.csv")
# Create figure
fig = go.Figure()
# Add surface trace
fig.add_trace(go.Heatmap(z=df.values.tolist(), colorscale="Viridis"))
# Update plot sizing
fig.update_layout(
width=800,
height=900,
autosize=False,
margin=dict(t=100, b=0, l=0, r=0),
)
# Update 3D scene options
fig.update_scenes(
aspectratio=dict(x=1, y=1, z=0.7),
aspectmode="manual"
)
# Add dropdowns
button_layer_1_height = 1.08
fig.update_layout(
updatemenus=[
dict(
buttons=list([
dict(
args=["colorscale", "Viridis"],
label="Viridis",
method="restyle"
),
dict(
args=["colorscale", "Cividis"],
label="Cividis",
method="restyle"
),
dict(
args=["colorscale", "Blues"],
label="Blues",
method="restyle"
),
dict(
args=["colorscale", "Greens"],
label="Greens",
method="restyle"
),
]),
direction="down",
pad={"r": 10, "t": 10},
showactive=True,
x=0.1,
xanchor="left",
y=button_layer_1_height,
yanchor="top"
),
dict(
buttons=list([
dict(
args=["reversescale", False],
label="False",
method="restyle"
),
dict(
args=["reversescale", True],
label="True",
method="restyle"
)
]),
direction="down",
pad={"r": 10, "t": 10},
showactive=True,
x=0.37,
xanchor="left",
y=button_layer_1_height,
yanchor="top"
),
dict(
buttons=list([
dict(
args=[{"contours.showlines": False, "type": "contour"}],
label="Hide lines",
method="restyle"
),
dict(
args=[{"contours.showlines": True, "type": "contour"}],
label="Show lines",
method="restyle"
),
]),
direction="down",
pad={"r": 10, "t": 10},
showactive=True,
x=0.58,
xanchor="left",
y=button_layer_1_height,
yanchor="top"
),
]
)
fig.update_layout(
annotations=[
dict(text="colorscale", x=0, xref="paper", y=1.06, yref="paper",
align="left", showarrow=False),
dict(text="Reverse<br>Colorscale", x=0.25, xref="paper", y=1.07,
yref="paper", showarrow=False),
dict(text="Lines", x=0.54, xref="paper", y=1.06, yref="paper",
showarrow=False)
])
fig.show()
import plotly.graph_objects as go
# Generate dataset
import numpy as np
np.random.seed(1)
x0 = np.random.normal(2, 0.4, 400)
y0 = np.random.normal(2, 0.4, 400)
x1 = np.random.normal(3, 0.6, 600)
y1 = np.random.normal(6, 0.4, 400)
x2 = np.random.normal(4, 0.2, 200)
y2 = np.random.normal(4, 0.4, 200)
# Create figure
fig = go.Figure()
# Add traces
fig.add_trace(
go.Scatter(
x=x0,
y=y0,
mode="markers",
marker=dict(color="DarkOrange")
)
)
fig.add_trace(
go.Scatter(
x=x1,
y=y1,
mode="markers",
marker=dict(color="Crimson")
)
)
fig.add_trace(
go.Scatter(
x=x2,
y=y2,
mode="markers",
marker=dict(color="RebeccaPurple")
)
)
# Add buttons that add shapes
cluster0 = [dict(type="circle",
xref="x", yref="y",
x0=min(x0), y0=min(y0),
x1=max(x0), y1=max(y0),
line=dict(color="DarkOrange"))]
cluster1 = [dict(type="circle",
xref="x", yref="y",
x0=min(x1), y0=min(y1),
x1=max(x1), y1=max(y1),
line=dict(color="Crimson"))]
cluster2 = [dict(type="circle",
xref="x", yref="y",
x0=min(x2), y0=min(y2),
x1=max(x2), y1=max(y2),
line=dict(color="RebeccaPurple"))]
fig.update_layout(
updatemenus=[
dict(buttons=list([
dict(label="None",
method="relayout",
args=["shapes", []]),
dict(label="Cluster 0",
method="relayout",
args=["shapes", cluster0]),
dict(label="Cluster 1",
method="relayout",
args=["shapes", cluster1]),
dict(label="Cluster 2",
method="relayout",
args=["shapes", cluster2]),
dict(label="All",
method="relayout",
args=["shapes", cluster0 + cluster1 + cluster2])
]),
)
]
)
# Update remaining layout properties
fig.update_layout(
title_text="Highlight Clusters",
showlegend=False,
)
fig.show()
Update Dropdown¶
The "update"
method should be used when modifying the data and layout sections of the graph.
This example demonstrates how to update which traces are displayed while simultaneously updating layout attributes such as the chart title and annotations.
import plotly.graph_objects as go
import pandas as pd
# Load dataset
df = pd.read_csv(
"https://raw.githubusercontent.com/plotly/datasets/master/finance-charts-apple.csv")
df.columns = [col.replace("AAPL.", "") for col in df.columns]
# Initialize figure
fig = go.Figure()
# Add Traces
fig.add_trace(
go.Scatter(x=list(df.Date),
y=list(df.High),
name="High",
line=dict(color="#33CFA5")))
fig.add_trace(
go.Scatter(x=list(df.Date),
y=[df.High.mean()] * len(df.index),
name="High Average",
visible=False,
line=dict(color="#33CFA5", dash="dash")))
fig.add_trace(
go.Scatter(x=list(df.Date),
y=list(df.Low),
name="Low",
line=dict(color="#F06A6A")))
fig.add_trace(
go.Scatter(x=list(df.Date),
y=[df.Low.mean()] * len(df.index),
name="Low Average",
visible=False,
line=dict(color="#F06A6A", dash="dash")))
# Add Annotations and Buttons
high_annotations = [dict(x="2016-03-01",
y=df.High.mean(),
xref="x", yref="y",
text="High Average:<br> %.3f" % df.High.mean(),
ax=0, ay=-40),
dict(x=df.Date[df.High.idxmax()],
y=df.High.max(),
xref="x", yref="y",
text="High Max:<br> %.3f" % df.High.max(),
ax=-40, ay=-40)]
low_annotations = [dict(x="2015-05-01",
y=df.Low.mean(),
xref="x", yref="y",
text="Low Average:<br> %.3f" % df.Low.mean(),
ax=0, ay=40),
dict(x=df.Date[df.High.idxmin()],
y=df.Low.min(),
xref="x", yref="y",
text="Low Min:<br> %.3f" % df.Low.min(),
ax=0, ay=40)]
fig.update_layout(
updatemenus=[
dict(
active=0,
buttons=list([
dict(label="None",
method="update",
args=[{"visible": [True, False, True, False]},
{"title": "Yahoo",
"annotations": []}]),
dict(label="High",
method="update",
args=[{"visible": [True, True, False, False]},
{"title": "Yahoo High",
"annotations": high_annotations}]),
dict(label="Low",
method="update",
args=[{"visible": [False, False, True, True]},
{"title": "Yahoo Low",
"annotations": low_annotations}]),
dict(label="Both",
method="update",
args=[{"visible": [True, True, True, True]},
{"title": "Yahoo",
"annotations": high_annotations + low_annotations}]),
]),
)
])
# Set title
fig.update_layout(title_text="Yahoo")
fig.show()
Reference¶
See https://plotly.com/python/reference/layout/updatemenus/ for more information about updatemenu
dropdowns.
What About Dash?¶
Dash is an open-source framework for building analytical applications, with no Javascript required, and it is tightly integrated with the Plotly graphing library.
Learn about how to install Dash at https://dash.plot.ly/installation.
Everywhere in this page that you see fig.show()
, you can display the same figure in a Dash application by passing it to the figure
argument of the Graph
component from the built-in dash_core_components
package like this:
import plotly.graph_objects as go # or plotly.express as px
fig = go.Figure() # or any Plotly Express function e.g. px.bar(...)
# fig.add_trace( ... )
# fig.update_layout( ... )
from dash import Dash, dcc, html
app = Dash()
app.layout = html.Div([
dcc.Graph(figure=fig)
])
app.run_server(debug=True, use_reloader=False) # Turn off reloader if inside Jupyter
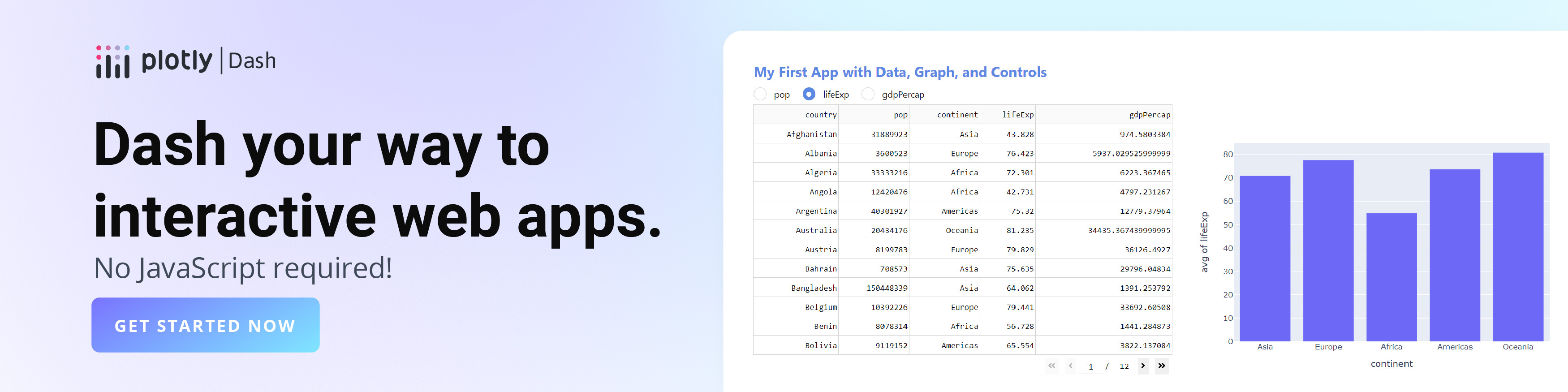